A decade ago, machine learning was everywhere. While the rise of generative AI has meant artificial intelligence has stolen the spotlight to some degree, it’s machine learning (ML) that silently powers its most impressive achievements.
From chatbots to self-driving cars, ML’s ability to analyse data, recognise patterns, and drive decisions forms the backbone of AI systems. At a recent visit to Half Stack London, Elastic's Carly Richmond showed off Is It Fake, a fun project inspired by the US TV programme Is It Cake? , with the site challenging users to distinguish between items that are real, and those made to look like everyday items when they are in fact made of cake.
This got us thinking about the role machine learning could play in future, in helping us distinguish between reality and fiction. Let’s dive into how this foundational technology is shaping our understanding of truth in a world increasingly blurred by artificial innovation.
What is Machine Learning?
Machine learning enables computers to identify patterns, make decisions, and improve over time by learning from data—without being explicitly programmed. This flexibility makes it indispensable across diverse applications, from fraud detection to creative content generation.
At its foundation, ML encompasses supervised, unsupervised, and reinforcement learning models, each tailored to distinct problems. The tools driving these capabilities - like TensorFlow and PyTorch among many others - have democratised access to AI, equipping developers and researchers with robust platforms to explore the boundaries of what's possible.
Recognising Fiction in a Digital World
In recent years we've seen the media landscape change, particularly in the realm of news and information. ML and AI have enhanced productivity, making it easier for us to create content of all kinds with less effort. However, it's also made it more challenging for us to tell real, legitimate information (or content) from the fabricated. With this in mind, let's consider a few ways that ML might actually help clear up the mess it contributed towards making.
Deepfake Detection
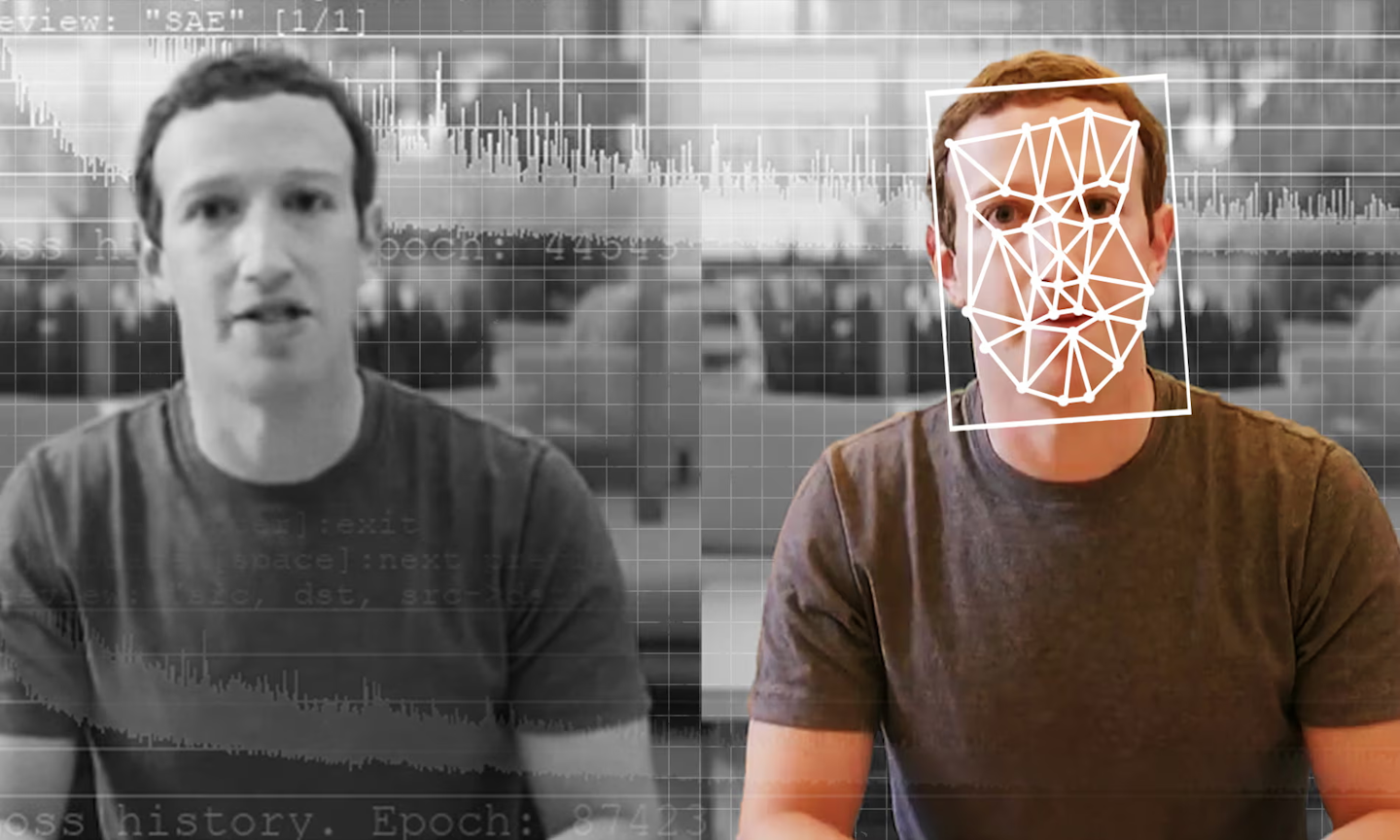
Deepfakes - AI-generated video and audio that convincingly mimic real individuals - pose significant ethical and security challenges. In just the last five years we've seen deep fakes developer from being obviously fake, to incredibly advanced and capable of fooling the majority of viewers. To counteract this, ML models are trained on datasets of real and synthesised media, identifying subtle artefacts like unnatural eye movement or inconsistencies in lighting.
For instance, Convolutional Neural Networks (CNNs) and Temporal Convolutional Networks (TCNs) have proven effective in analysing pixel-level discrepancies and temporal irregularities in video frames. In a world where political battles are increasing fought online, these kinds of tools will likely become essential for us to tell the difference between real and fake video content.
AI-Generated Text Identification
With tools like ChatGPT, GPT-4, and Bard producing increasingly human-like text, distinguishing between human-written and machine-generated content has become critical. Researchers use ML techniques to analyse linguistic patterns, sentence structure, and context coherence to flag AI-produced content. Natural Language Processing (NLP) models, fine-tuned on diverse datasets, excel in spotting these nuances.
Authenticity in Art and Media
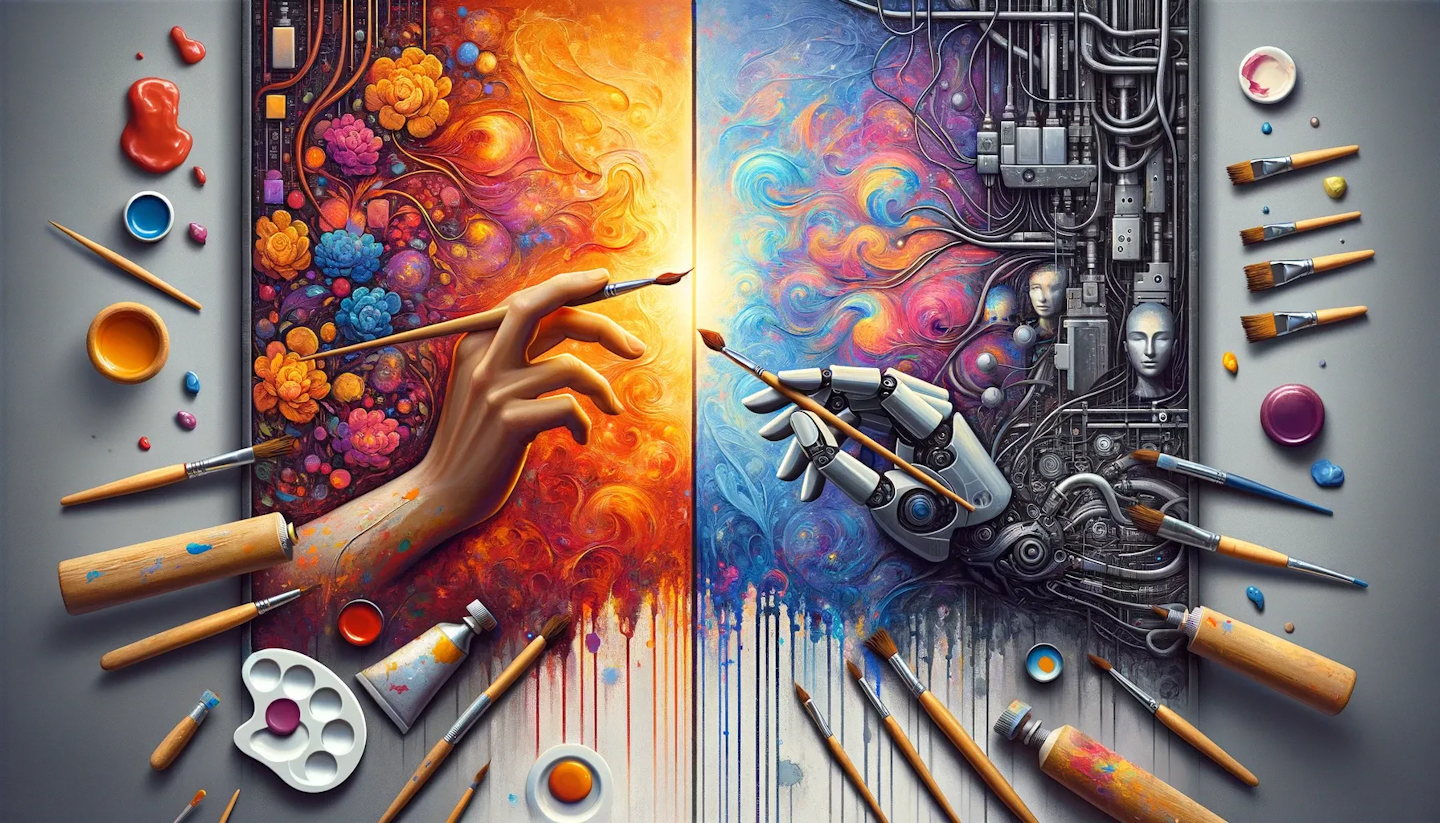
The rise of AI-generated art (think DALL·E, MidJourney, Grok, Bing Image Creator) prompts questions about authorship and originality. Models designed to authenticate artworks often analyse brushstroke patterns, colour usage, and compositional consistency to distinguish human creativity from creative content made by computers.
Of course we can't predict the future, but it's fair to suggest that in future we might categorise artwork made by AI separately to that made by humans, as the human touch is always appreciated by those using or consuming the creative content.
Gaming and Virtual Reality
As immersive virtual environments blur the line between simulation and reality, ML is being applied to differentiate user-driven actions from scripted events or bots. These insights are vital for ensuring fairness in competitive gaming and safeguarding virtual experiences from manipulation.
Why It Matters
Machine learning is becoming essential in tackling the growing challenge of separating reality from fiction in today’s digital world.
It helps combat misinformation by detecting fake media before it spreads, building trust in what we see online and protecting people (and organisations) from harm.
In an age where the value of original creative content is under threat, ML promotes fair, ethical content creation by making AI-generated outputs more transparent and respecting intellectual property. As synthetic content becomes harder to spot, ML is critical to staying one step ahead.
As ML advances, its application in recognising fiction within the digital world highlights a fascinating convergence of technology, ethics, and creativity.
Whether its detecting deepfakes, authenticating digital art, analysing AI-generated text, the uses of machine learning cannot be overstated in terms of its importance in society, both now and in the future.
If you enjoyed this article, we recommend you go and check out Carly Richmond - Senior Developer Advocate at Elastic - discussing how she used machine learning and TensorFlow.js to create Is It (F)ake?.
Visit the watch section now to watch Carly's session from HalfStack London now.
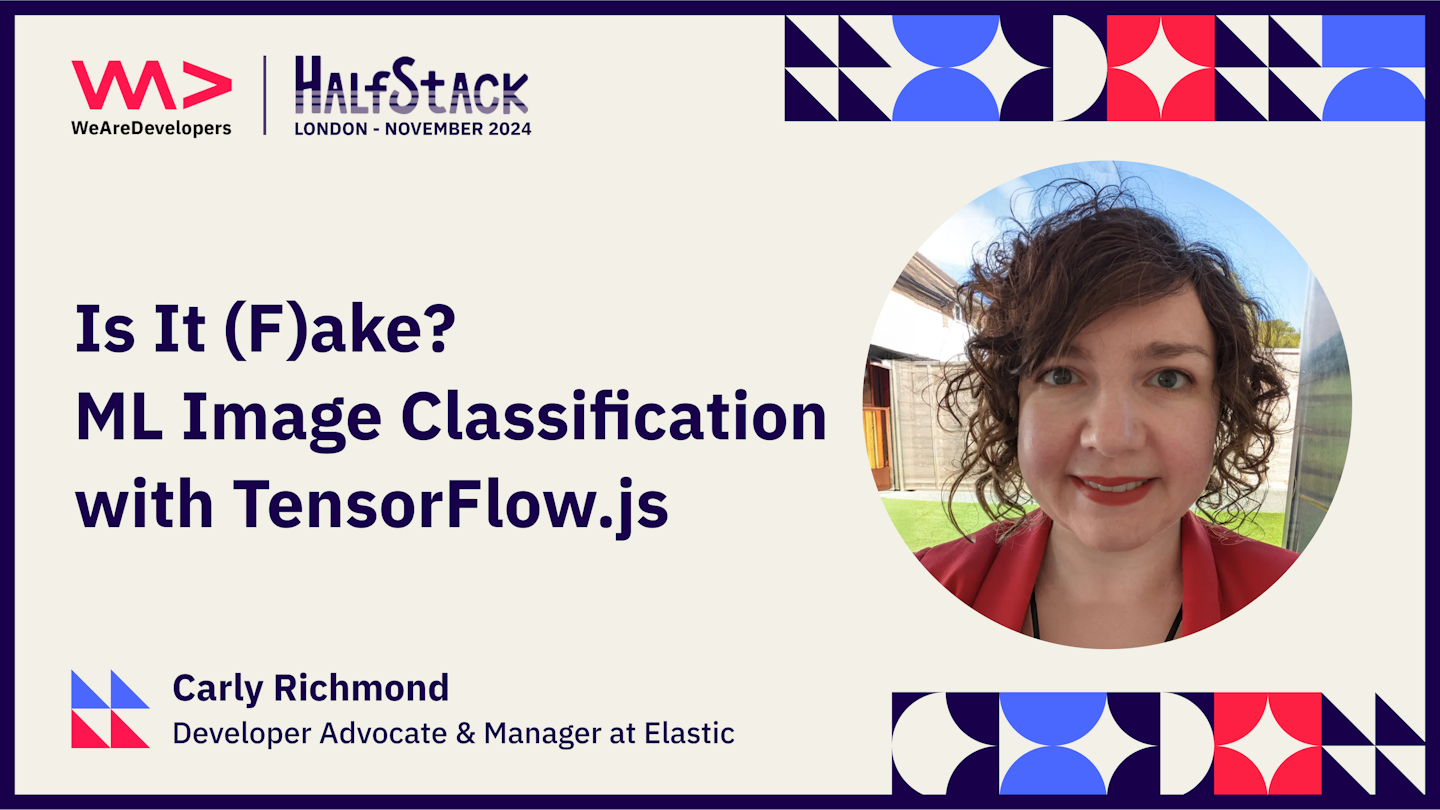